R
R
R
R
R
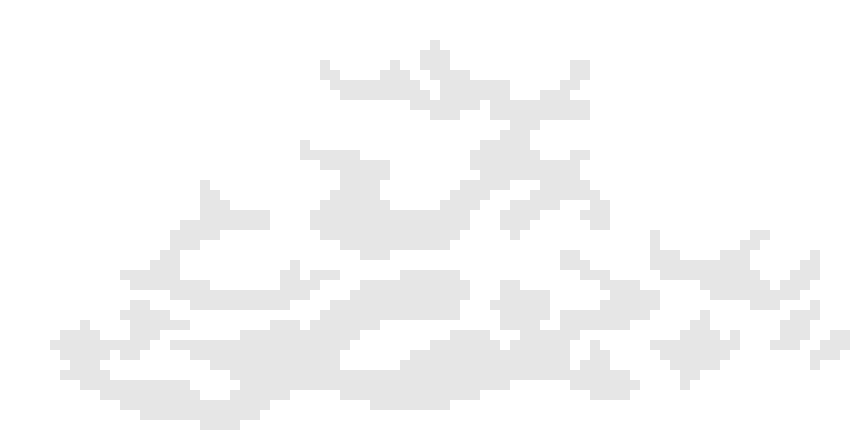
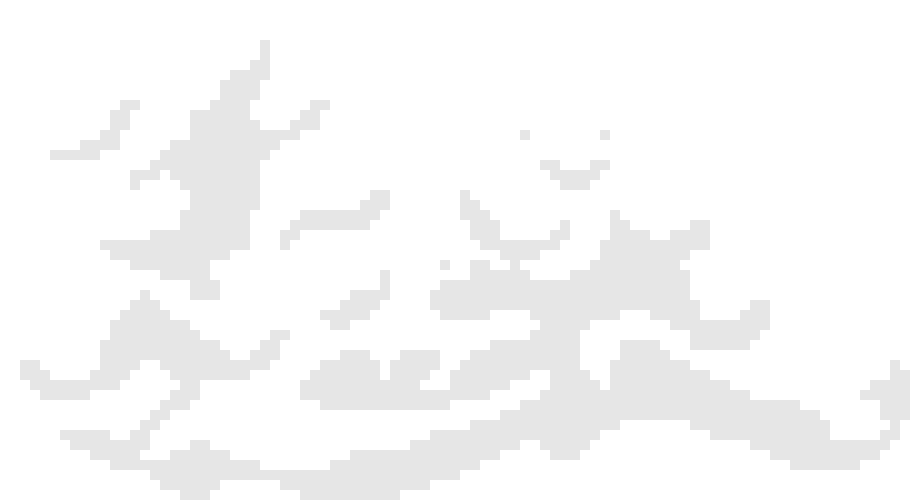
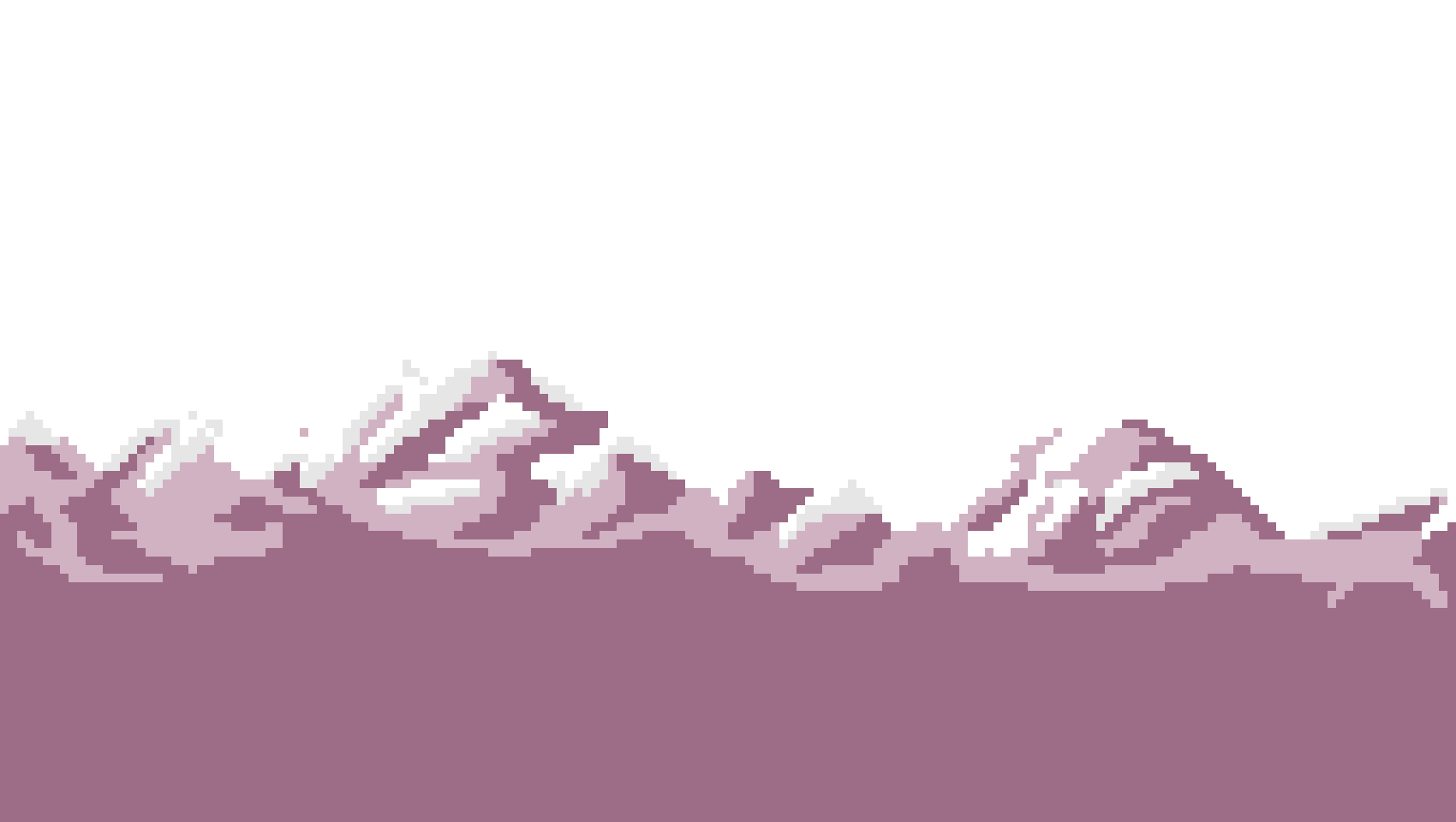
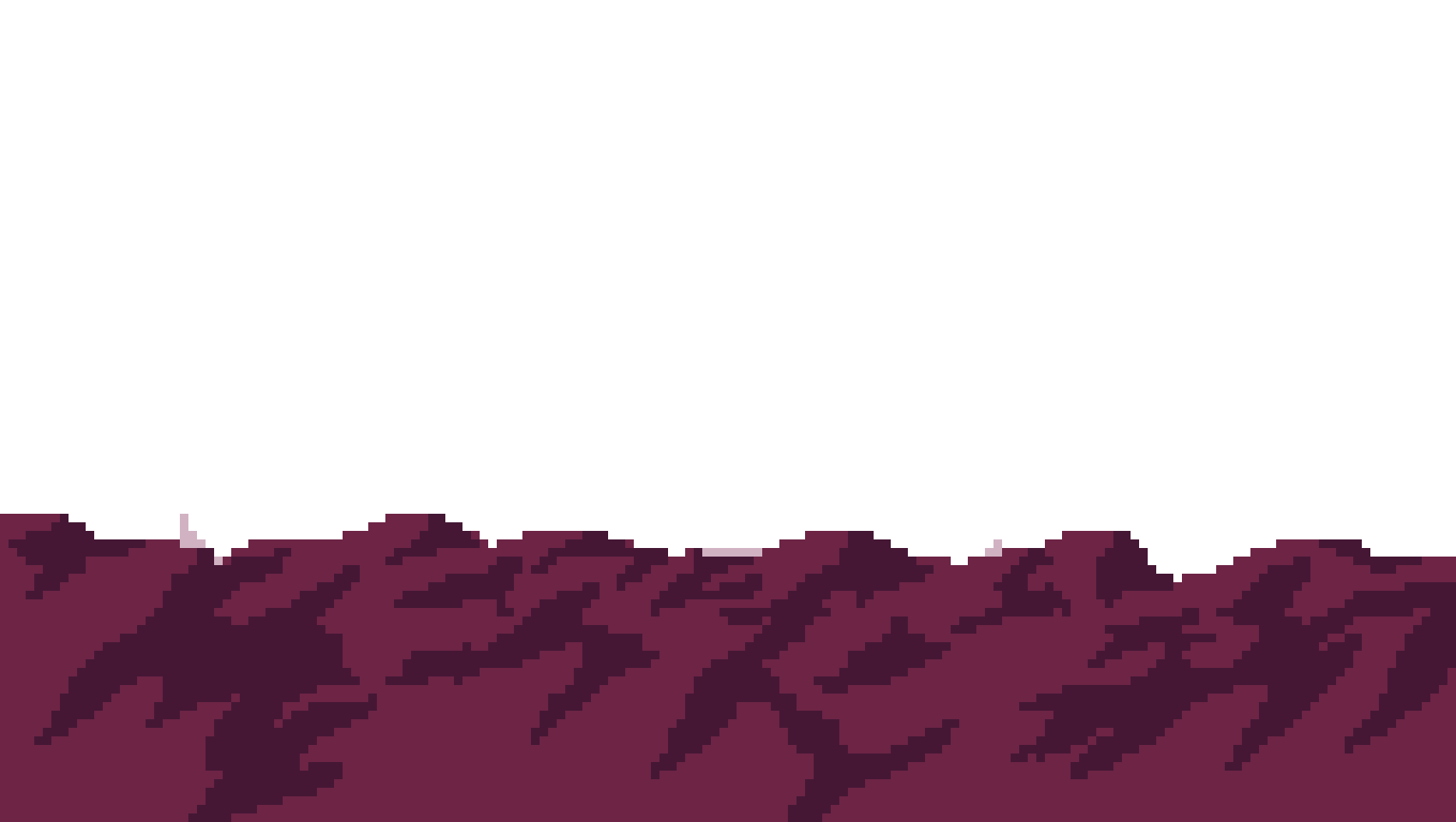
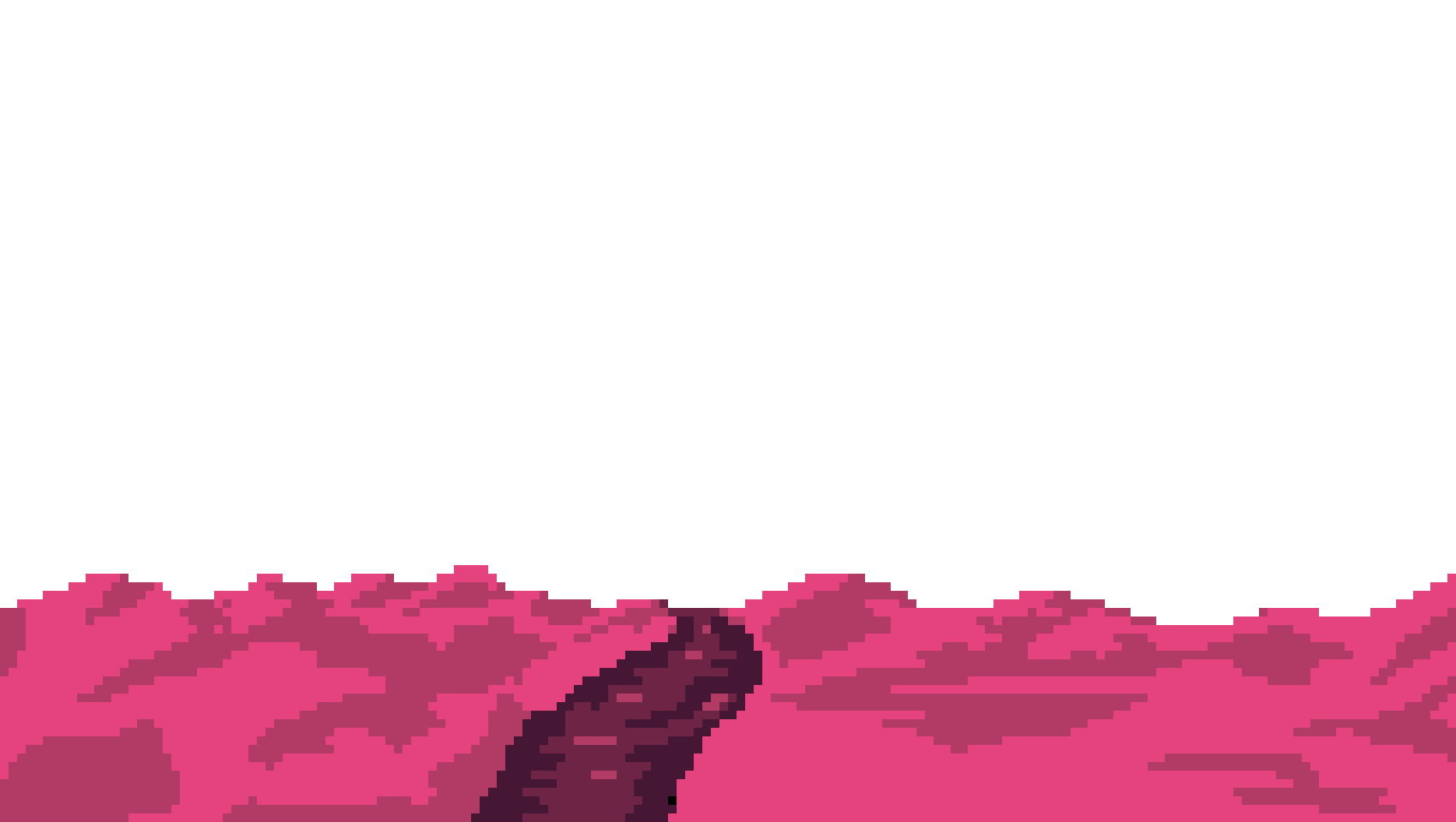
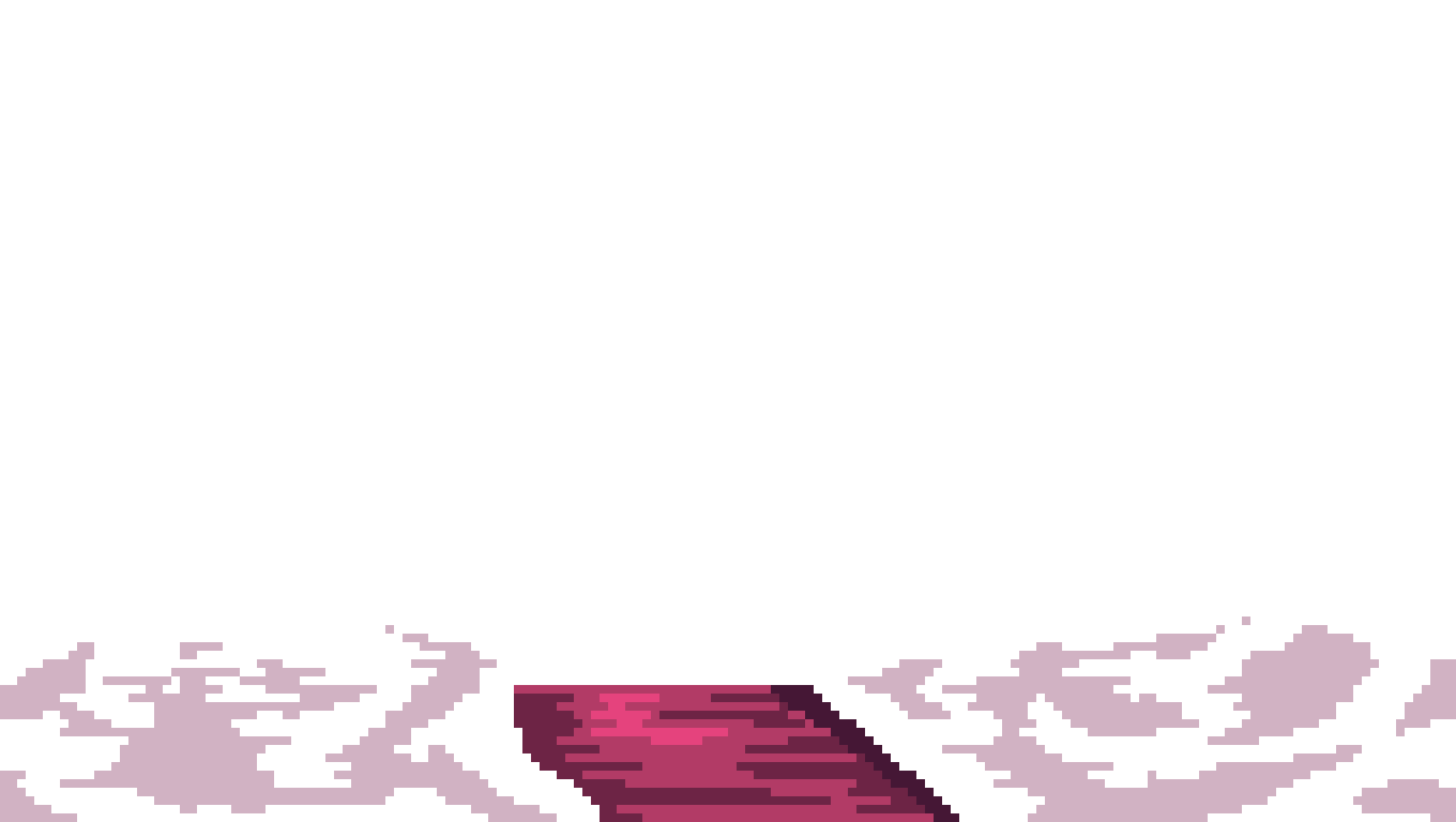
Countermeasures
Machine learning can help reduce toxicity, improving online conversation
“AI holds the potential for some of the biggest advances we are going to see.”
-Sundar Pichai
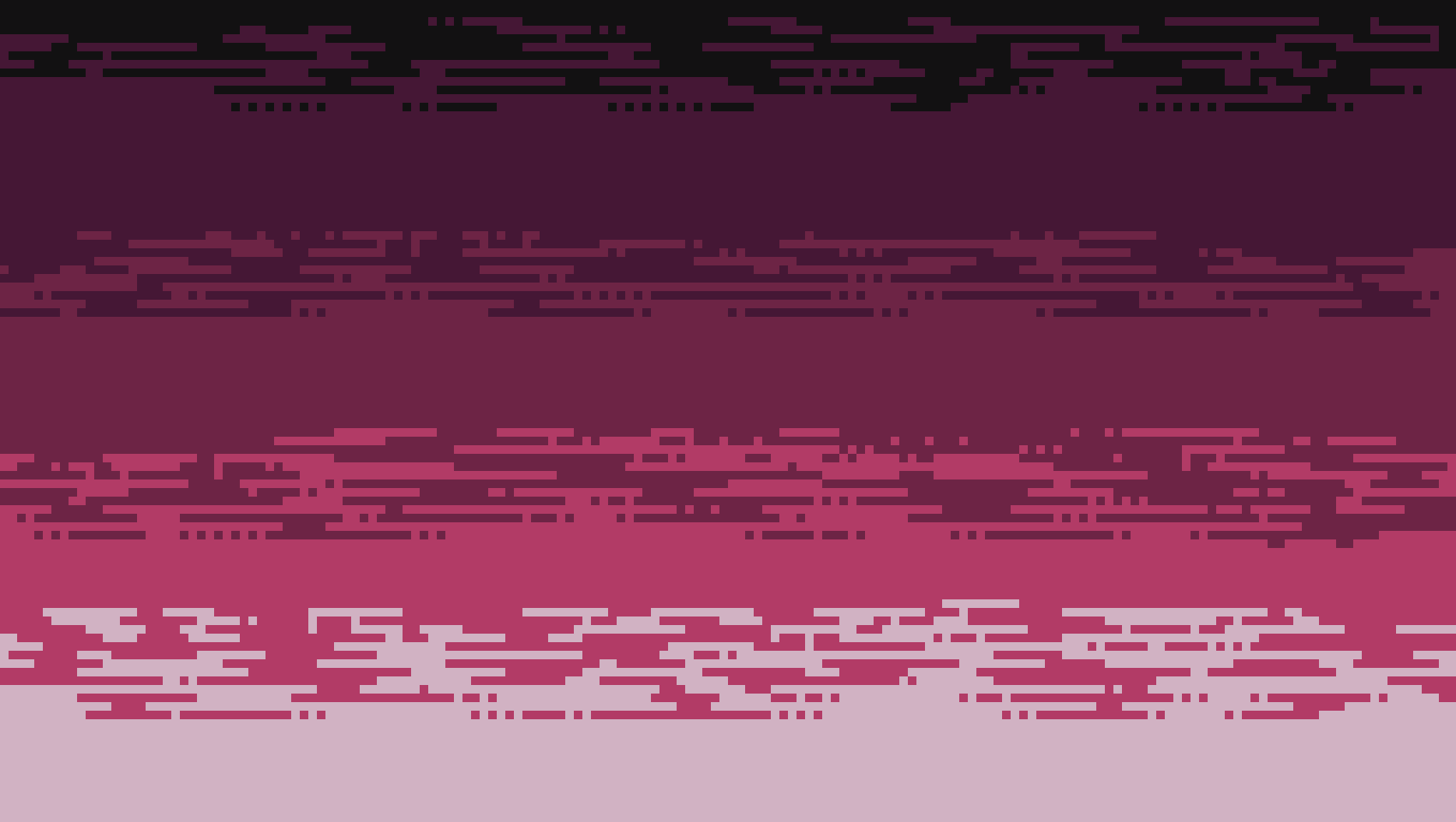
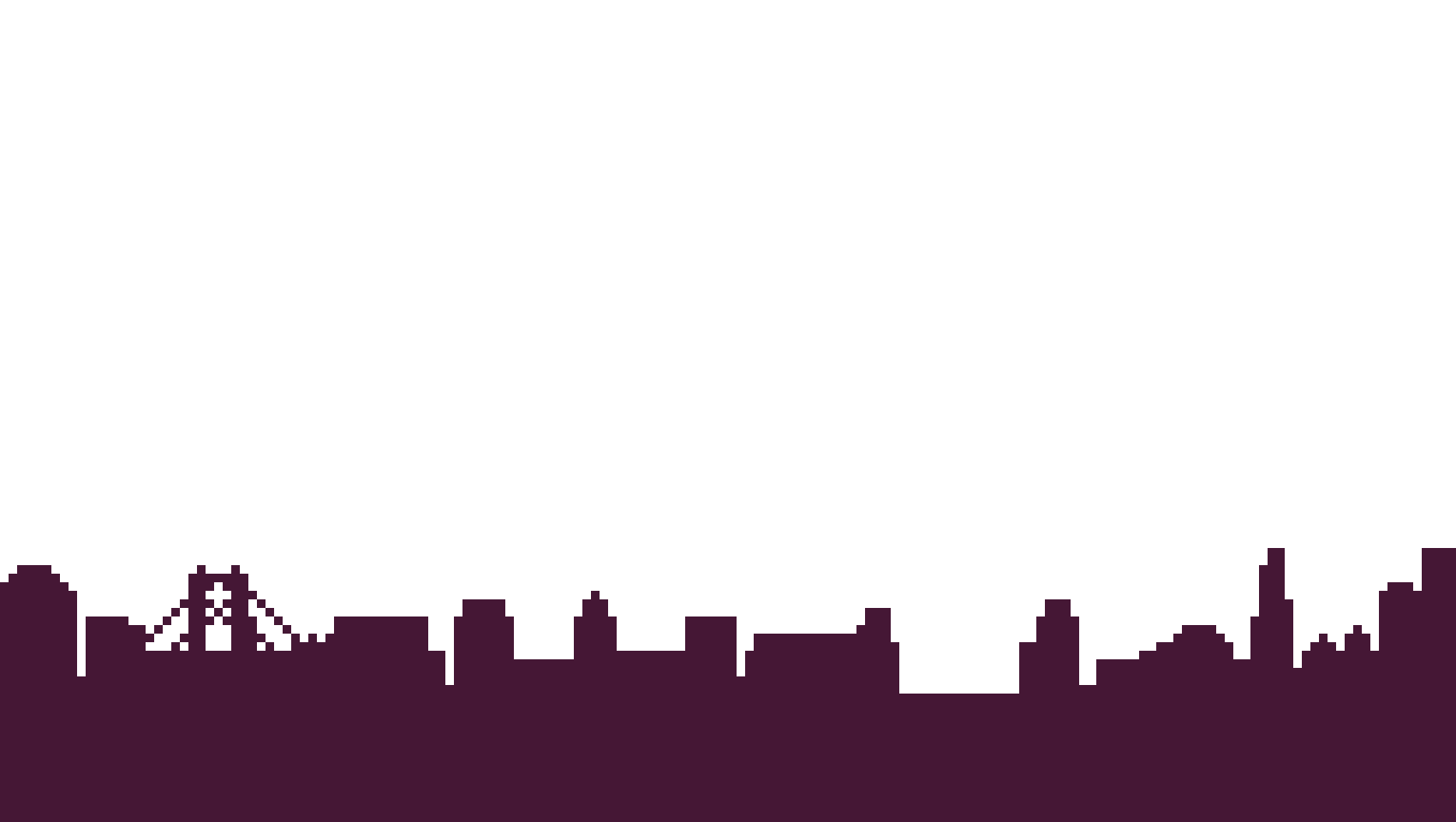
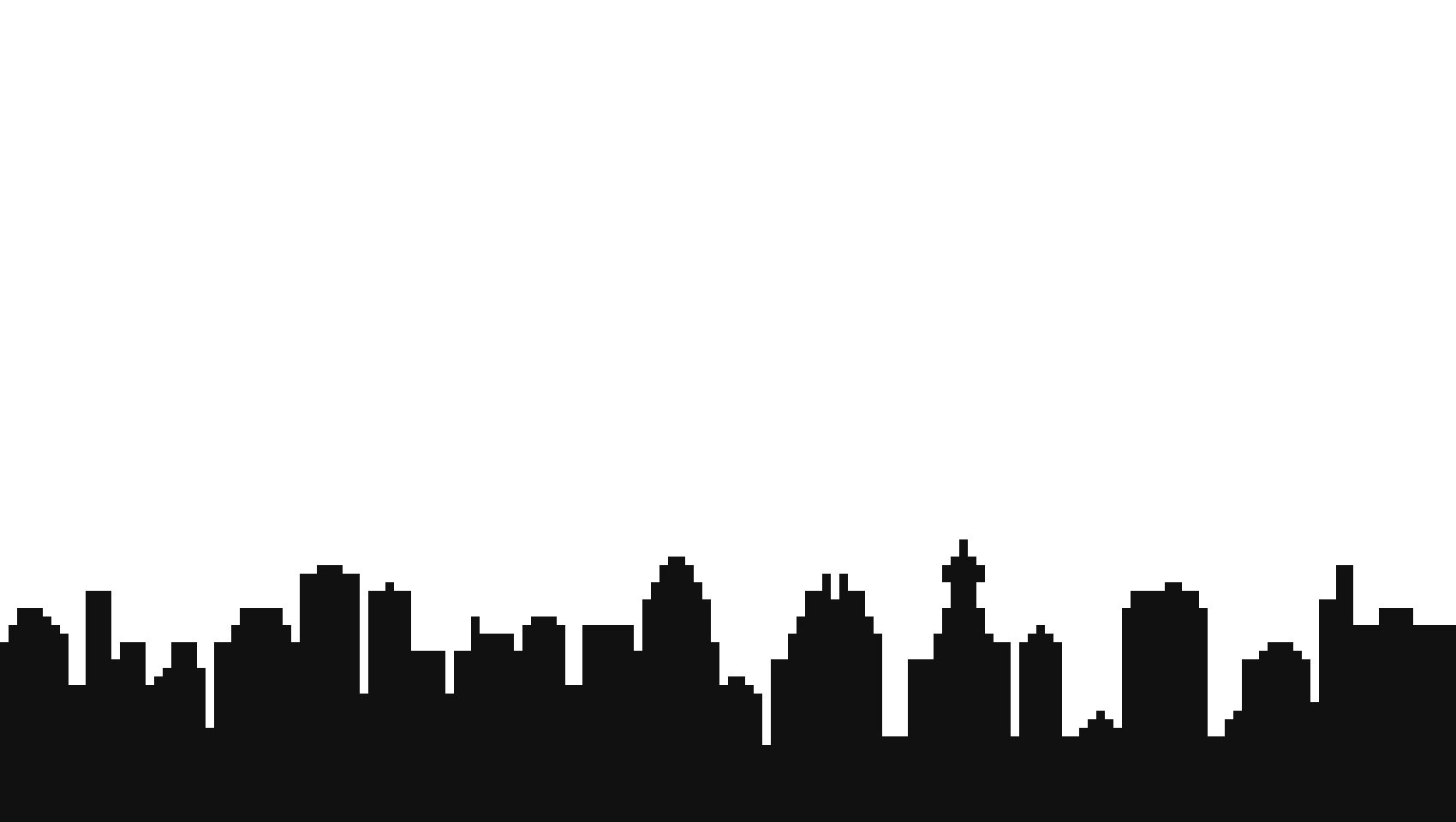
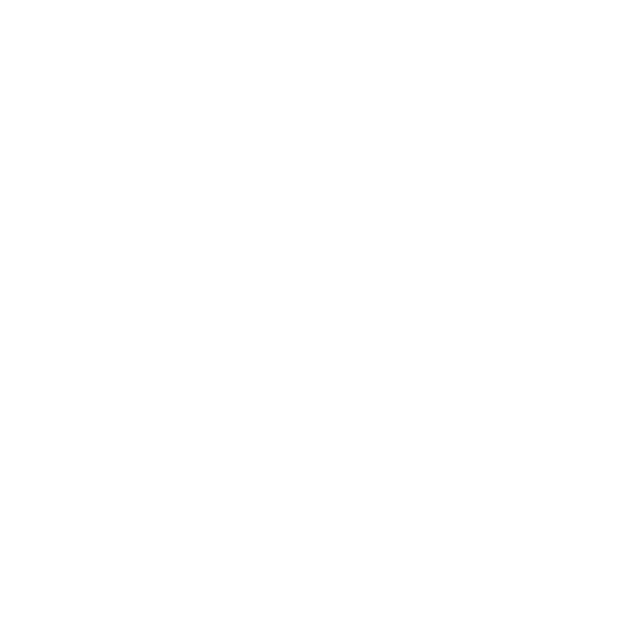
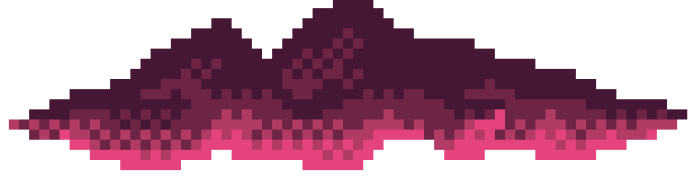
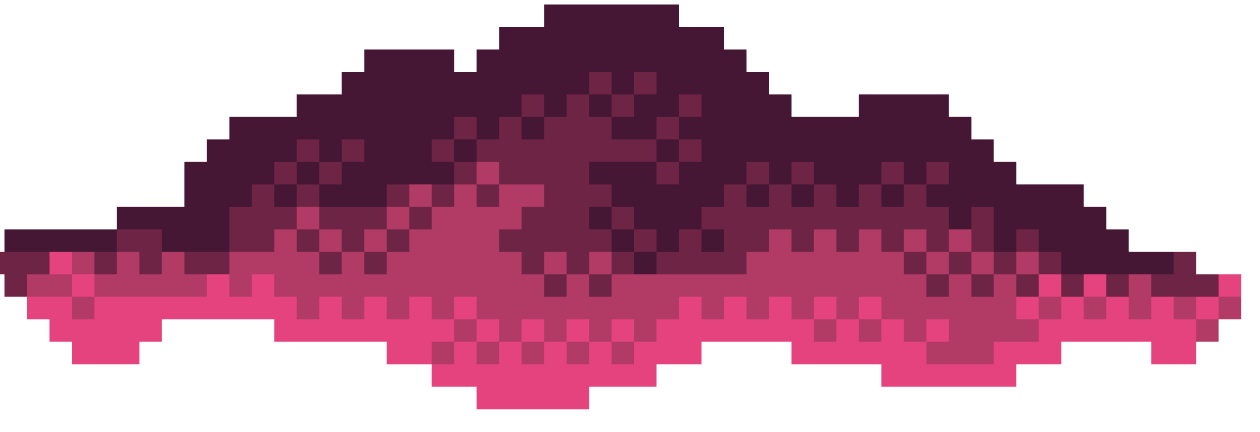
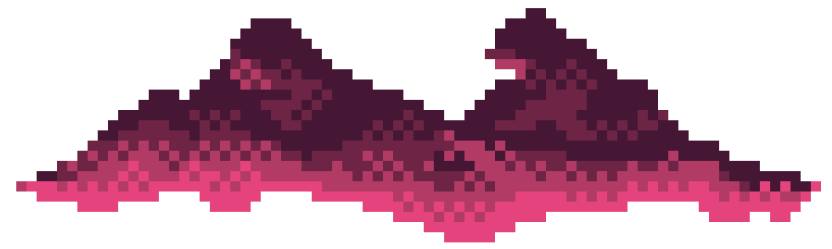
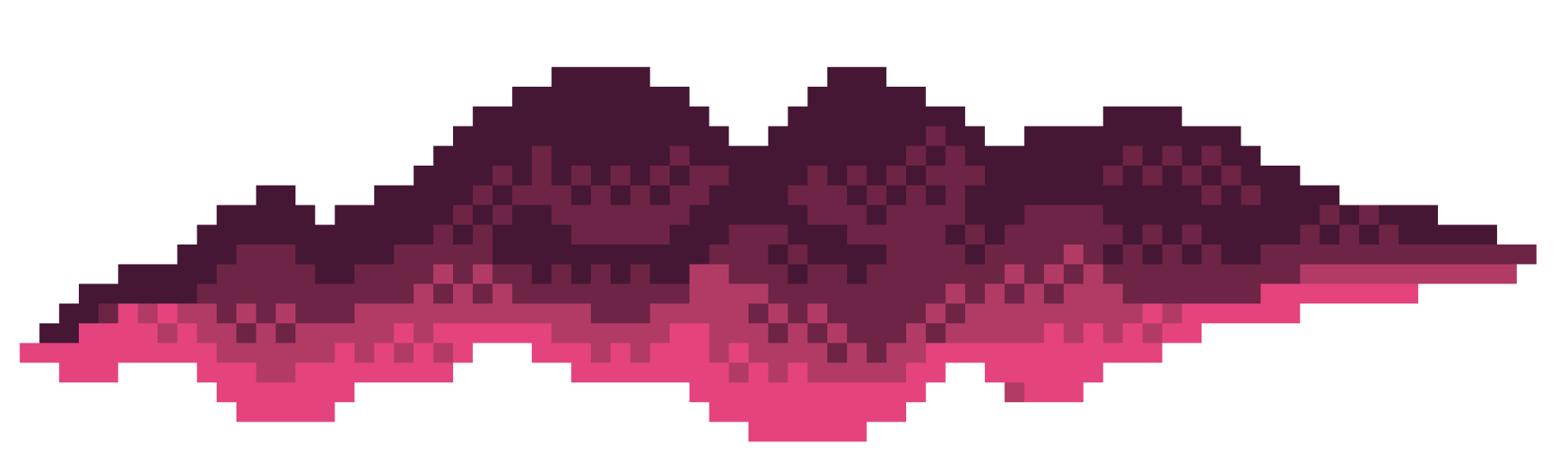
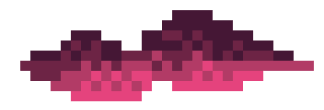
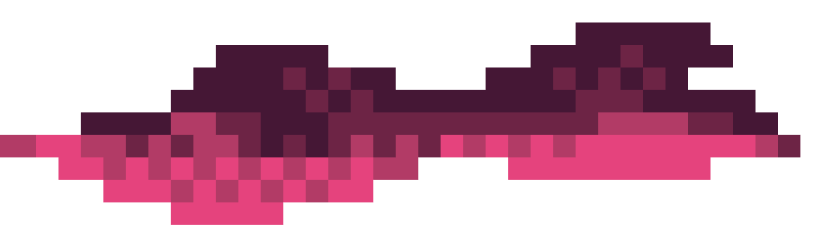
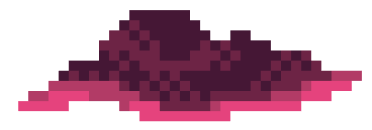
Meet Perspective
Perspective is a free API that uses machine learning to spot toxic comments by scoring their perceived impact on conversation.
We built Perspective using models that we developed in partnership with Google’s Counter Abuse Technology team. The training process is as follows:
Gather data from the internet
The team gathered millions of comments. A group of content moderators and annotators labelled each comment for whether or not they felt the comment was toxic.
Train and test the models
Models were trained on this dataset and learned to predict toxicity based on the examples in the dataset. The models were tested in a variety of scenarios to validate that they learned the right associations, and then were released for use with publishers and platforms.
Use feedback to improve
Publishers and platforms using the models provide feedback and expand the dataset. Models are frequently retrained to make improvements and keep them up-to-date.
Empowering publishers to create more space for dialogue
“Working with Perspective has enabled us to encourage positive interactions across hundreds of dynamic and engaged communities and tens of millions of users. The solutions are elegant and frictionless, supporting our vision for higher quality engagement with less toxicity.”
-Nadav Shoval, CEO & Co-Founder OpenWeb


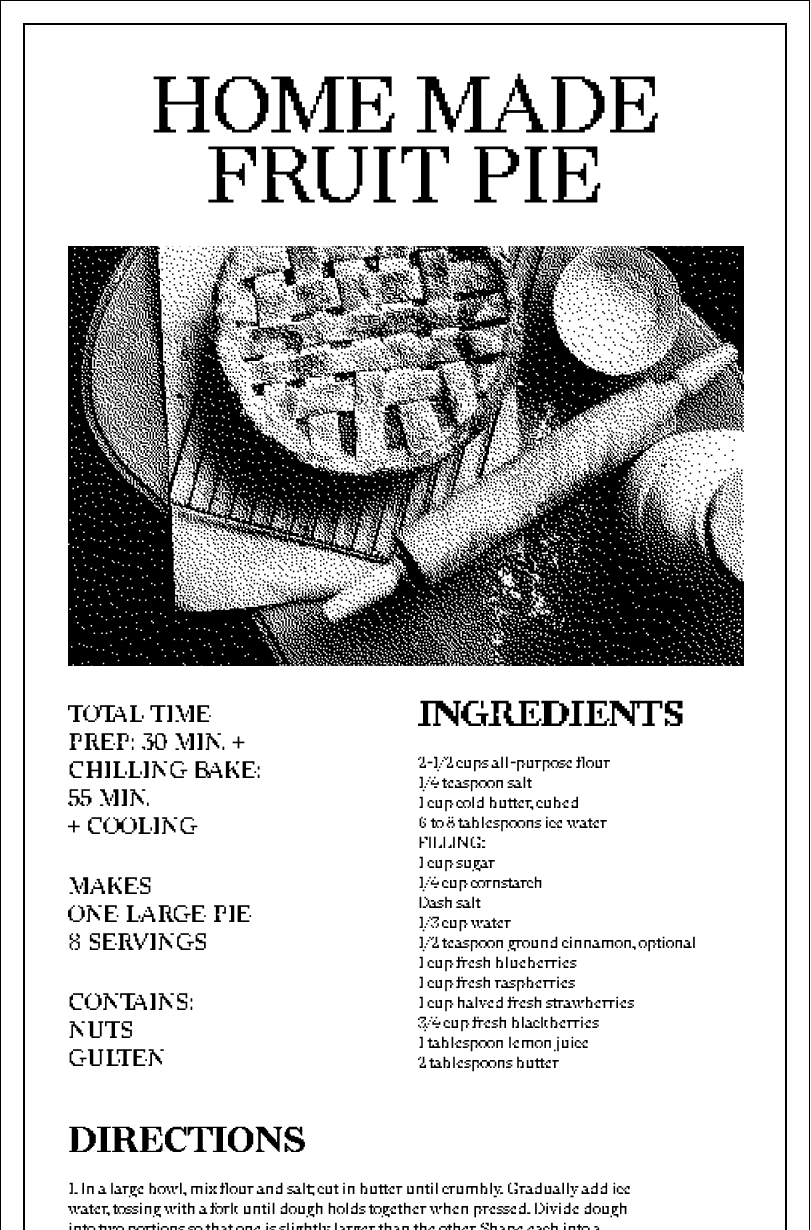
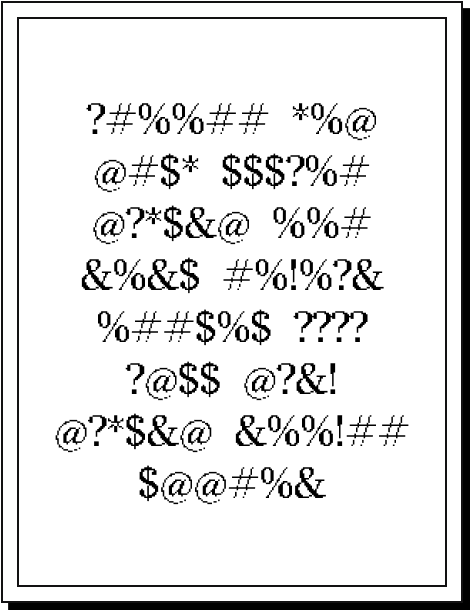
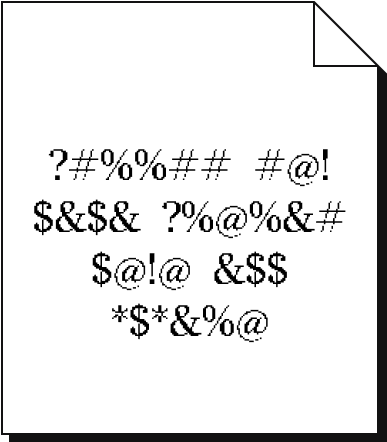
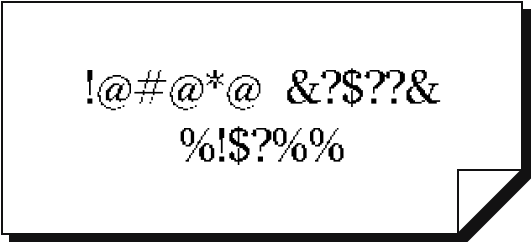
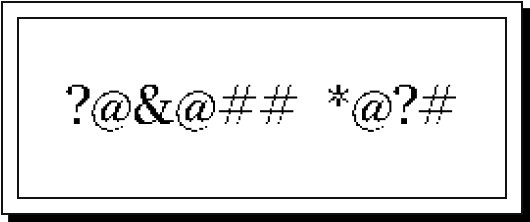
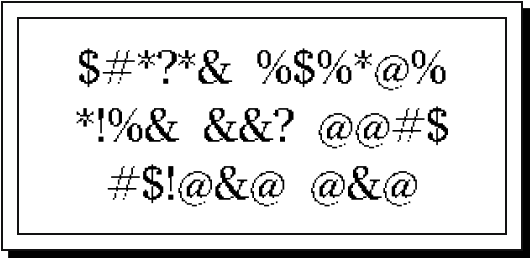
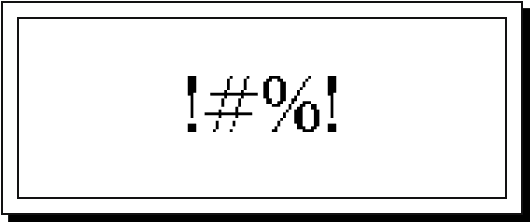
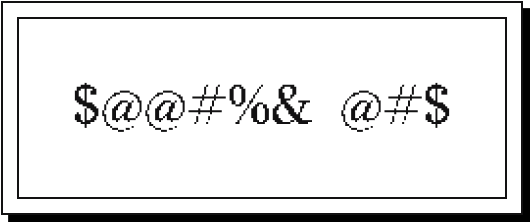
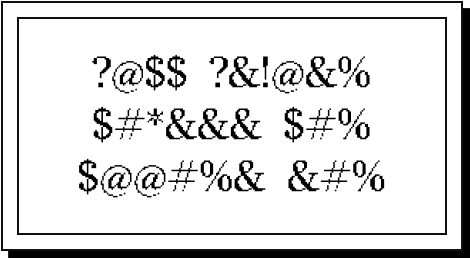
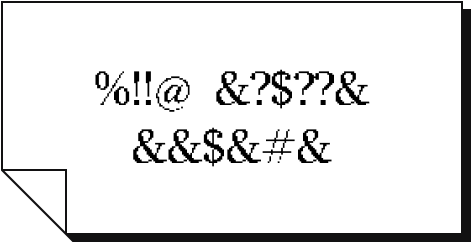
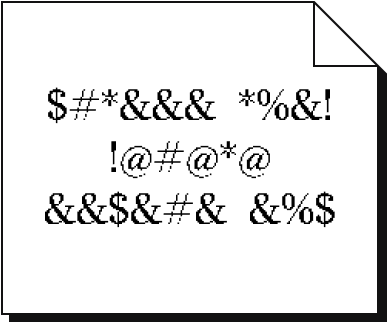
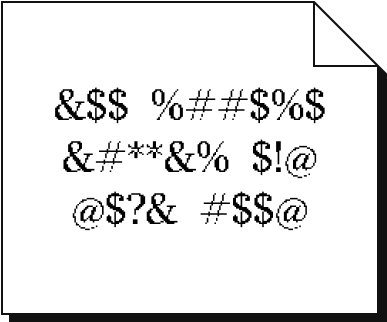

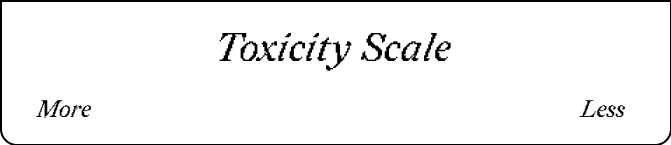
Perspective processes over
requests every day
Perspective has a demonstrated ability to reduce toxicity on a diverse range of platforms, from major publishers like The New York Times and The Wall Street Journal to small newspapers like the Southeast Missourian, to online gaming platforms like FACEIT. In each case, data suggests that using Perspective can measurably increase participation and reduce toxicity, all while reducing the burden on the human resources required to moderate platforms.
Machine learning models are subject to human biases
M achine learning can measurably reduce toxicity online, but the technology is far from perfect. Perspective still makes mistakes and, just like any machine learning model, is subject to the biases of the world that created it.
What is algorithmic bias?
Algorithmic bias is the phenomena of observing results that are systematically prejudiced. Humans and the real world have biases, and when we build models from data from the real world, we risk propagating or amplifying that bias. When those flawed assumptions are built into a model, the output of the model is inherently flawed, too.
Understanding and addressing bias in Perspective
After the initial launch of Perspective API in 2017, users discovered a positive correlation between identity terms containing information on race or sexual orientation and toxicity score. For example, the phrase "I am a gay Black woman" received a high toxicity score. In this case, the identity terms are not being used pejoratively, so this example was classified incorrectly.
The source of the error was the training data itself—the training set did not contain sufficient examples of nontoxic comments containing identity terms for the model to learn that the terms themselves were neutral. This was because the vast majority of usage of these words in online forums is toxic—the model was truly reflecting the state of the world. But the context of that usage matters.
Challenges like this illuminate opportunities for improvement to machine learning. In fact, mitigating bias in data and algorithms is a primary area of ongoing research for Perspective API. This problem is not unique to Perspective API. Further research is needed to make the role played by AI and ML in content moderation processes more transparent. Many of our partners are committed to exploring ways to mitigate bias in machine learning.
“We’re committed to constantly improving our models and to having open, transparent conversations about model performance with those impacted by our models.”
-Lucy Vasserman, Software Engineer at Jigsaw
Building Perspective is an ongoing process of discovery and improvement. Our hope that being open and sharing our research and experiences will help the whole industry improve together as we work to create less biased, fairer models for everyone.
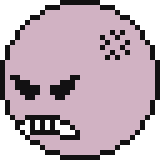
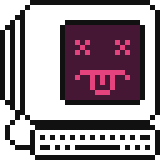
See Perspective in action
Explore the issue: